About
Maintained by
Fengwei Wang, Hui Zhu, Rongxing Lu, Yandong Zheng, and Hao Li
Xidian University
266 Xinglong Section of Xifeng Road, Xi'an, Shaanxi 710126, China
Abstract
It is believed that online disease risk assessment system has great potential to alleviate the medical treatment problems for the future smart city, as it can excavate disease risk factors from a large number of patient features, providing diagnostic references for doctors and saving medical treatment time for patients. However, due to the sensitivity of medical data, the flourish of online disease risk assessment service still faces severe challenges including information privacy and security. In this paper, based on naive bayesian classification, we propose an efficient and privacy-preserving disease risk assessment scheme over multi-party outsourced vertical datasets, named CARER. With CARER, the e-healthcare provider can securely aggregate vertically distributed medical data from multiple medical centers (i.e., hospitals) for training a global disease risk prediction model, and provide privacy-preserving disease risk assessment services for users (i.e., patients and doctors). Specifically, during the data gregation and disease risk query phases, all sensitive data are operated over ciphertexts without decryption. Therefore, the private information of medical centers, e-healthcare provider, and users can be well protected. Detailed security analysis shows that CARER can resist various known security threats. In addition, we implement CARER with real medical datasets, and extensive simulation results demonstrate that CARER is efficient and can be implemented effectively.
Conceptual Architecture of Online Disease Risk Assessment Service
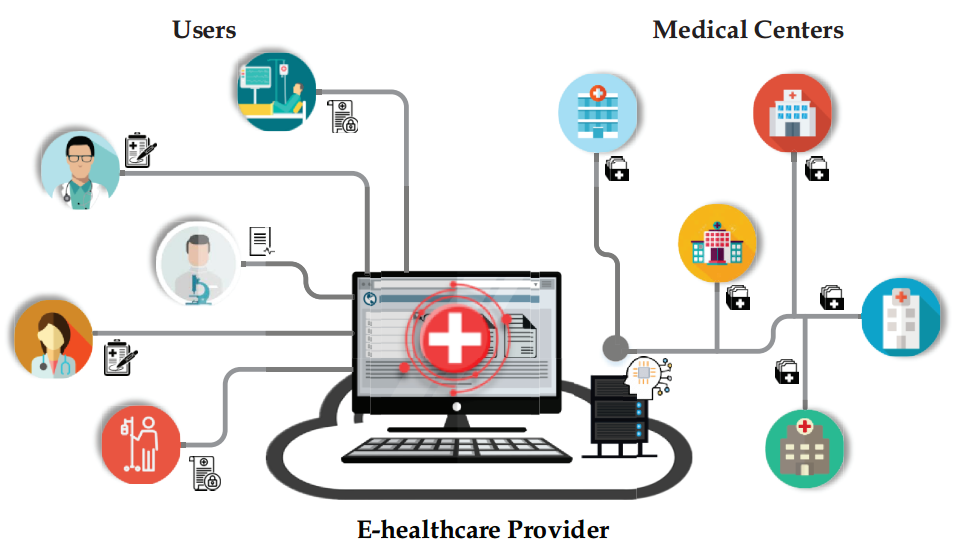
System Model Under Considered
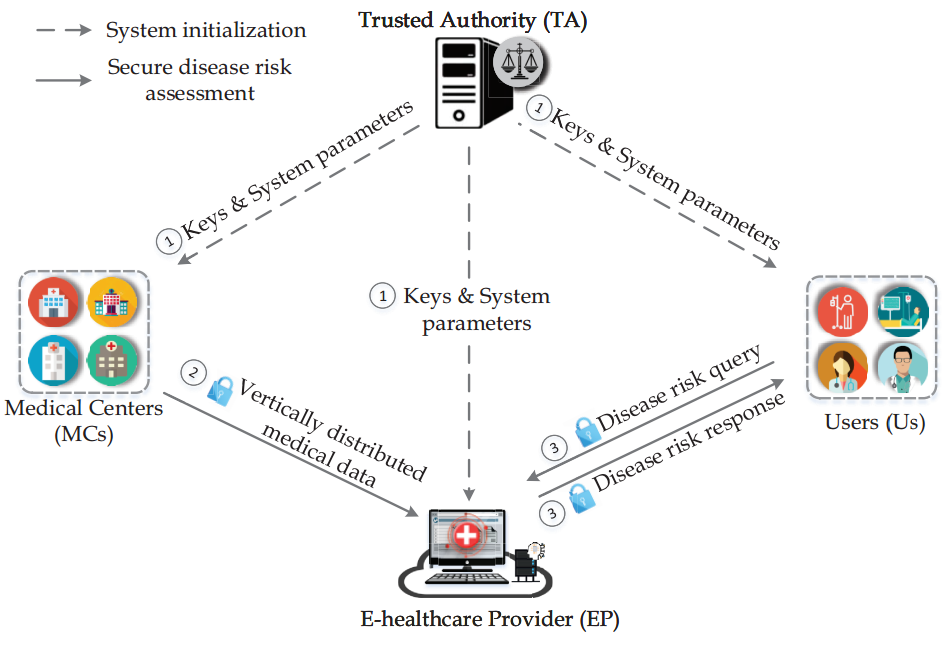
Overview of Carer
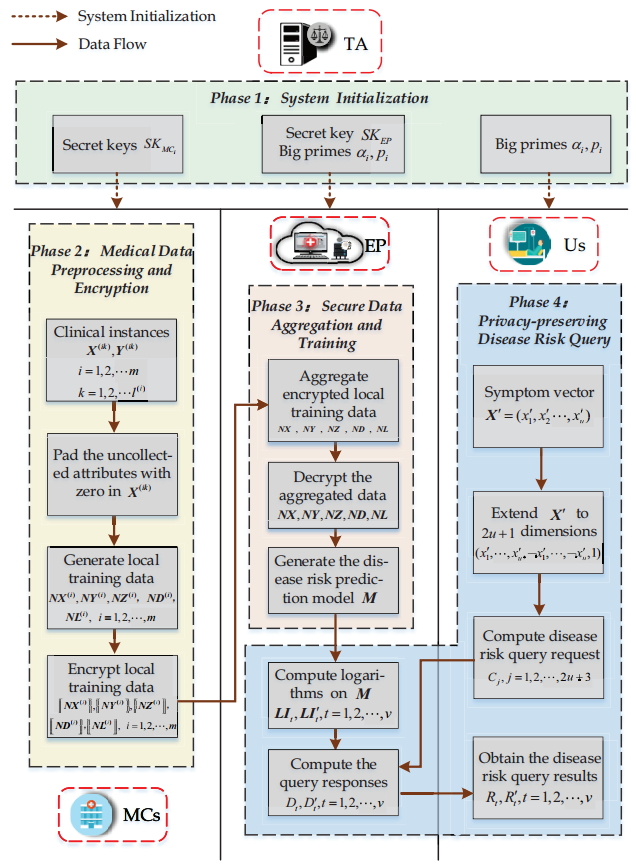
Computation Cost of Carer
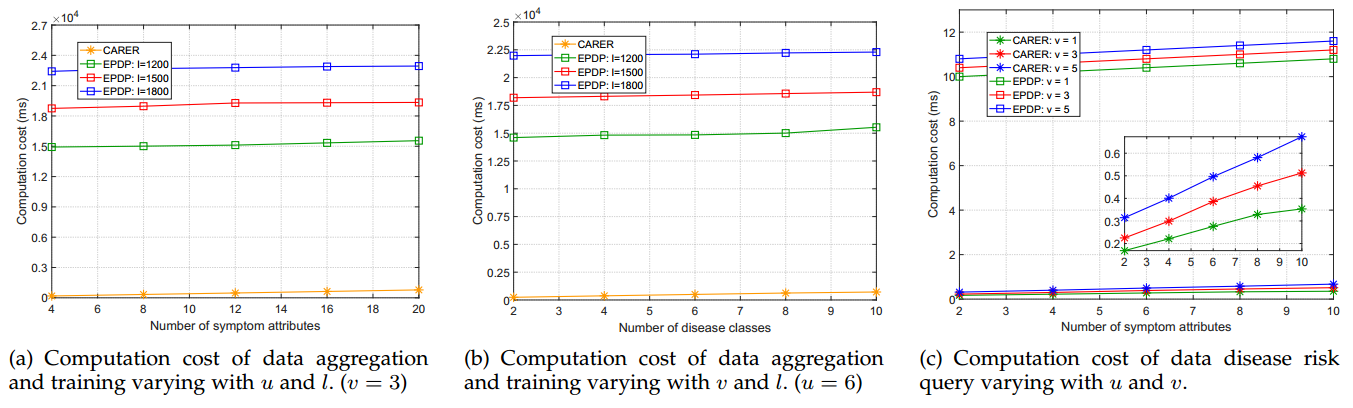
Communication Overhead of Carer
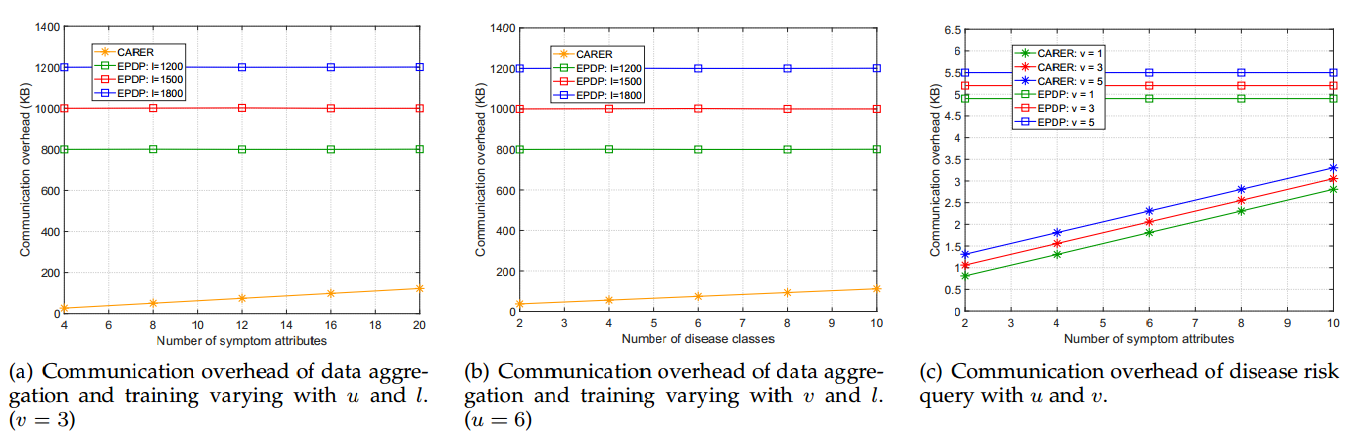